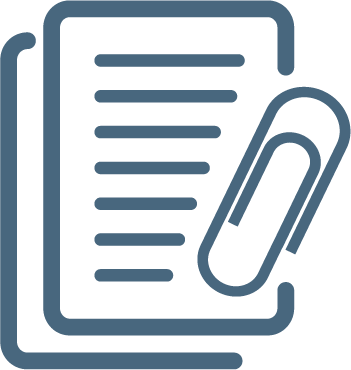
Journal of the Royal Statistical Society. Series B: Statistical Methodology
1467-9868
1369-7412
Anh Quốc
Cơ quản chủ quản: OXFORD UNIV PRESS , Wiley-Blackwell Publishing Ltd
Phân tích ảnh hưởng
Thông tin về tạp chí
1467-9868
1369-7412
Anh Quốc
Cơ quản chủ quản: OXFORD UNIV PRESS , Wiley-Blackwell Publishing Ltd