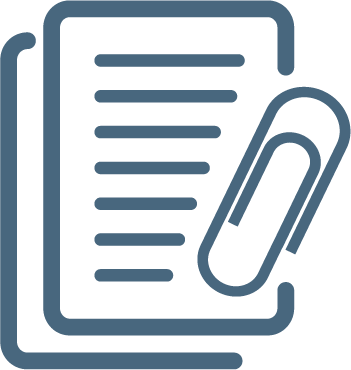
Machine Learning
1573-0565
0885-6125
Cơ quản chủ quản: Springer Netherlands , SPRINGER
Lĩnh vực:
SoftwareArtificial Intelligence
Các bài báo tiêu biểu
Learning in the presence of concept drift and hidden contexts
Tập 23 - Trang 69-101 - 1996
On-line learning in domains where the target concept depends on some hidden context poses serious problems. A changing context can induce changes in the target concepts, producing what is known as concept drift. We describe a family of learning algorithms that flexibly react to concept drift and can take advantage of situations where contexts reappear. The general approach underlying all these algorithms consists of (1) keeping only a window of currently trusted examples and hypotheses; (2) storing concept descriptions and reusing them when a previous context re-appears; and (3) controlling both of these functions by a heuristic that constantly monitors the system's behavior. The paper reports on experiments that test the systems' perfomance under various conditions such as different levels of noise and different extent and rate of concept drift.
Competition-based induction of decision models from examples
Tập 13 - Trang 229-257 - 1993
Symbolic induction is a promising approach to constructing decision models by extracting regularities from a data set of examples. The predominant type of model is a classification rule (or set of rules) that maps a set of relevant environmental features into specific categories or values. Classifying loan risk based on borrower profiles, consumer choice from purchase data, or supply levels based on operating conditions are all examples of this type of model-building task. Although current inductive approaches, such as ID3 and CN2, perform well on certain problems, their potential is limited by the incremental nature of their search. Genetic algorithms (GA) have shown great promise on complex search domains, and hence suggest a means for overcoming these limitations. However, effective use of genetic search in this context requires a framework that promotes the fundamental model-building objectives of predictive accuracy and model simplicity. In this article we describe COGIN, a GA-based inductive system that exploits the conventions of induction from examples to provide this framework. The novelty of COGIN lies in its use of training set coverage to simultaneously promote competition in various classification niches within the model and constrain overall model complexity. Experimental comparisons with NewID and CN2 provide evidence of the effectiveness of the COGIN framework and the viability of the GA approach.
Erratum to: Preference Relation-based Markov Random Fields for Recommender Systems
Tập 106 - Trang 547-547 - 2017
Entropic risk minimization for nonparametric estimation of mixing distributions
Tập 99 - Trang 119-136 - 2014
We discuss a nonparametric estimation method for the mixing distributions in mixture models. The problem is formalized as a minimization of a one-parameter objective functional, which becomes the maximum likelihood estimation or the kernel vector quantization in special cases. Generalizing the theorem for the nonparametric maximum likelihood estimation, we prove the existence and discreteness of the optimal mixing distribution and provide an algorithm to calculate it. It is demonstrated that with an appropriate choice of the parameter, the proposed method is less prone to overfitting than the maximum likelihood method. We further discuss the connection between the unifying estimation framework and the rate-distortion problem.
Efficient Approximations for the Marginal Likelihood of Bayesian Networks with Hidden Variables
Tập 29 - Trang 181-212 - 1997
We discuss Bayesian methods for model averaging and model selection among Bayesian-network models with hidden variables. In particular, we examine large-sample approximations for the marginal likelihood of naive-Bayes models in which the root node is hidden. Such models are useful for clustering or unsupervised learning. We consider a Laplace approximation and the less accurate but more computationally efficient approximation known as the Bayesian Information Criterion (BIC), which is equivalent to Rissanen's (1987) Minimum Description Length (MDL). Also, we consider approximations that ignore some off-diagonal elements of the observed information matrix and an approximation proposed by Cheeseman and Stutz (1995). We evaluate the accuracy of these approximations using a Monte-Carlo gold standard. In experiments with artificial and real examples, we find that (1) none of the approximations are accurate when used for model averaging, (2) all of the approximations, with the exception of BIC/MDL, are accurate for model selection, (3) among the accurate approximations, the Cheeseman–Stutz and Diagonal approximations are the most computationally efficient, (4) all of the approximations, with the exception of BIC/MDL, can be sensitive to the prior distribution over model parameters, and (5) the Cheeseman–Stutz approximation can be more accurate than the other approximations, including the Laplace approximation, in situations where the parameters in the maximum a posteriori configuration are near a boundary.
Dynamic Parameter Encoding for Genetic Algorithms
Tập 9 - Trang 9-21 - 1992
The common use of static binary place-value codes for real-valued parameters of the phenotype in Holland's genetic algorithm (GA) forces either the sacrifice of representational precision for efficiency of search or vice versa. Dynamic Parameter Encoding (DPE) is a mechanism that avoids this dilemma by using convergence statistics derived from the GA population to adaptively control the mapping from fixed-length binary genes to real values. DPE is shown to be empirically effective and amenable to analysis; we explore the problem of premature convergence in GAs through two convergence models.
Recovering networks from distance data
Tập 92 - Trang 251-283 - 2013
A fully probabilistic approach to reconstructing Gaussian graphical models from distance data is presented. The main idea is to extend the usual central Wishart model in traditional methods to using a likelihood depending only on pairwise distances, thus being independent of geometric assumptions about the underlying Euclidean space. This extension has two advantages: the model becomes invariant against potential bias terms in the measurements, and can be used in situations which on input use a kernel- or distance matrix, without requiring direct access to the underlying vectors. The latter aspect opens up a huge new application field for Gaussian graphical models, as network reconstruction is now possible from any Mercer kernel, be it on graphs, strings, probabilities or more complex objects. We combine this likelihood with a suitable prior to enable Bayesian network inference. We present an efficient MCMC sampler for this model and discuss the estimation of module networks. Experiments depict the high quality and usefulness of the inferred networks.
Bayesian object matching
Tập 92 - Trang 225-250 - 2013
Matching of object refers to the problem of inferring unknown co-occurrence or alignment between observations or samples in two data sets. Given two sets of equally many samples, the task is to find for each sample a representative sample in the other set, without prior knowledge on a distance measure between the sets. Given a distance measure, the problem would correspond to a linear assignment problem, the problem of finding a permutation that re-orders samples in one set to minimize the total distance. When no such measure is available, we need to consider more complex solutions. Typical approaches maximize statistical dependency between the two sets, whereas in this work we present a Bayesian solution that builds a joint model for the two sources. We learn a Bayesian canonical correlation analysis model that includes a permutation parameter for re-ordering the samples in one of the sets. We provide both variational and sampling-based inference for approximative Bayesian analysis, and demonstrate on three data sets that the resulting methods outperform the earlier solutions.
Bagging Equalizes Influence
Tập 55 - Trang 251-270 - 2004
Bagging constructs an estimator by averaging predictors trained on bootstrap samples. Bagged estimates almost consistently improve on the original predictor. It is thus important to understand the reasons for this success, and also for the occasional failures. It is widely believed that bagging is effective thanks to the variance reduction stemming from averaging predictors. However, seven years from its introduction, bagging is still not fully understood. This paper provides experimental evidence supporting the hypothesis that bagging stabilizes prediction by equalizing the influence of training examples. This effect is detailed in two different frameworks: estimation on the real line and regression. Bagging’s improvements/deteriorations are explained by the goodness/badness of highly influential examples, in situations where the usual variance reduction argument is at best questionable. Finally, reasons for the equalization effect are advanced. They support that other resampling strategies such as half-sampling should provide qualitatively identical effects while being computationally less demanding than bootstrap sampling.
On the Discrepancy between Kleinberg’s Clustering Axioms and k-Means Clustering Algorithm Behavior
Tập 112 - Trang 2501-2553 - 2023
This paper performs an investigation of Kleinberg’s axioms (from both an intuitive and formal standpoint) as they relate to the well-known k-mean clustering method. The axioms, as well as a novel variations thereof, are analyzed in Euclidean space. A few natural properties are proposed, resulting in k-means satisfying the intuition behind Kleinberg’s axioms (or, rather, a small, and natural variation on that intuition). In particular, two variations of Kleinberg’s consistency property are proposed, called centric consistency and motion consistency. It is shown that these variations of consistency are satisfied by k-means.