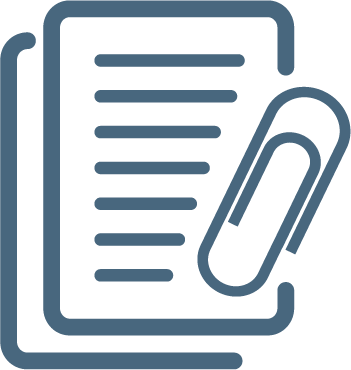
Association for Computing Machinery (ACM)
1931-0145
1931-0153
Cơ quản chủ quản: N/A
Lĩnh vực:
Các bài báo tiêu biểu
Phần mềm khai thác dữ liệu WEKA Dịch bởi AI Đã hơn mười hai năm trôi qua kể từ khi WEKA được phát hành công khai lần đầu tiên. Trong thời gian đó, phần mềm đã được viết lại hoàn toàn từ đầu, phát triển mạnh mẽ và hiện nay đi kèm với một tài liệu về khai thác dữ liệu [35]. Hiện tại, WEKA được chấp nhận rộng rãi trong cả lĩnh vực học thuật và kinh doanh, có một cộng đồng năng động, và đã được tải xuống hơn 1.4 triệu lần kể từ khi được... ... hiện toàn bộ
Tập 11 Số 1 - Trang 10-18 - 2009
Nghiên cứu về hành vi của một số phương pháp cân bằng dữ liệu huấn luyện máy học Dịch bởi AI Có nhiều khía cạnh có thể ảnh hưởng đến hiệu suất đạt được bởi các hệ thống học hiện tại. Đã có báo cáo rằng một trong những khía cạnh này liên quan đến sự mất cân bằng lớp, trong đó các ví dụ trong dữ liệu huấn luyện thuộc về một lớp nào đó vượt trội số lượng so với các ví dụ thuộc lớp khác. Trong tình huống này, xảy ra trong dữ liệu thế giới thực mô tả một sự kiện hiếm nhưng quan trọng, ... ... hiện toàn bộ
Tập 6 Số 1 - Trang 20-29 - 2004
A survey of kernels for structured dataKernel methods in general and support vector machines in particular have been successful in various learning tasks on data represented in a single table. Much 'real-world' data, however, is structured - it has no natural representation in a single table. Usually, to apply kernel methods to 'real-world' data, extensive pre-processing is performed to embed the data into areal vector space an... ... hiện toàn bộ
Tập 5 Số 1 - Trang 49-58 - 2003
Outlier ensemblesEnsemble analysis is a widely used meta-algorithm for many data mining problems such as classification and clustering. Numerous ensemble-based algorithms have been proposed in the literature for these problems. Compared to the clustering and classification problems, ensemble analysis has been studied in a limited way in the outlier detection literature. In some cases, ensemble analysis tec... ... hiện toàn bộ
Tập 14 Số 2 - Trang 49-58 - 2013
Probabilistic logic learningThe past few years have witnessed an significant interest in probabilistic logic learning, i.e. in research lying at the intersection of probabilistic reasoning, logical representations, and machine learning. A rich variety of different formalisms and learning techniques have been developed. This paper provides an introductory survey and overview of the state-of-the-art in probabilistic lo... ... hiện toàn bộ
Tập 5 Số 1 - Trang 31-48 - 2003
Intelligent Disaster Response via Social Media Analysis A SurveyThe success of a disaster relief and response process is largely dependent on timely and accurate information regarding the status of the disaster, the surrounding environment, and the a ected people. This information is primarily provided by rst responders on-site and can be enhanced by the firsthand reports posted in real-time on social media. Many tools and methods have been developed t... ... hiện toàn bộ
Tập 19 Số 1 - Trang 46-59 - 2017
Meta-clustering of gene expression data and literature-based information
The current tendency in the life sciences to spawn ever growing amounts of high-throughput assays has led to a situation where the interpretation of data and the formulation of hypotheses lag the pace at which information is produced. Although the first generation of statistical algorithms scrutinizing single, large-scale data sets found their way into the biological community... ... hiện toàn bộ
Tập 5 Số 2 - Trang 101-112 - 2003