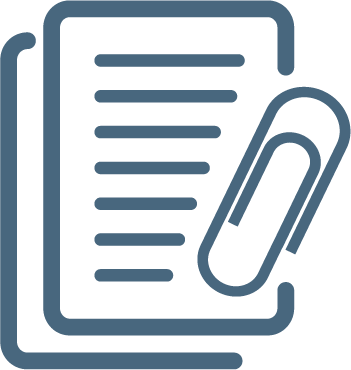
Neural Computing and Applications
1433-3058
0941-0643
Cơ quản chủ quản: SPRINGER LONDON LTD , Springer London
Lĩnh vực:
Artificial IntelligenceSoftware
Phân tích ảnh hưởng
Thông tin về tạp chí
Các bài báo tiêu biểu
Contracting and timing for outsourcing of information system with uncertain requirements
Tập 35 - Trang 2279-2289 - 2022
This research examined the impacts of market conditions on the choice of contract and timing for information system outsourcing. Real options approach is applied to develop several analytical models to investigate the decision making for outsourcing information system. The results show that the information asymmetry, requirement uncertainty, cost structure and vendor’s competition have important impacts on the client’s cost reduction, value of outsourcing option and probability of outsourcing. With symmetric information, the cost reduction, value of outsourcing option and probability of outsourcing under cost-plus contract and fixed-price contract are indifferent. With asymmetric information, however, the two contracts will generate different cost reductions and values of outsourcing option. We identify market conditions under which a contract is superior to others and also characterize the market conditions under which the client can switch to outsourcing or postpone outsourcing.
Factorized weight interaction neural networks for sparse feature prediction
Tập 32 - Trang 9567-9579 - 2019
Non-contiguous and categorical sparse feature data are widely existed on the Internet. To build a machine learning system with these data, it is important to properly model the interaction among features. In this paper, we propose a factorized weight interaction neural network (INN) with a new network structure called weight-interaction layer to learn patterns from feature interactions and factorized weight parameters of each feature interaction. The proposed INN can greatly reduce the dimension of sparse data via the weight-interaction layer, while the multi-layer neural network can be used to capture high-order feature latent patterns. Our experimental results on two real datasets show that the proposed method is able to effectively improve the prediction accuracy and generalization performance of the model, and consistently outperform related methods to be compared.
Global asymptotic and exponential synchronization of ring neural network with reaction–diffusion term and unbounded delay
- 2016
In this paper, we consider a ring neural network of coupled neurons with distributed and discrete time-varying delays along with the reaction–diffusion terms. We derive sufficient conditions that ensure the existence and uniqueness of the equilibrium point, synchronized asymptotic stability and exponential synchronization by using the theory of topological degree, properties of M-matrix, Lyapunov functional and analytic methods. The obtained results remove the assumption on the boundedness of activation functions. At the end, we give two examples to show the validity of our analysis.
Accelerated gradient learning algorithm for neural network weights update
Tập 19 - Trang 219-225 - 2009
This work proposes decomposition of square approximation algorithm for neural network weights update. Suggested improvement results in alternative method that converge in less iteration and is inherently parallel. Decomposition enables parallel execution convenient for implementation on computer grid. Improvements are reflected in accelerated learning rate which may be essential for time critical decision processes. Proposed solution is tested and verified on multilayer perceptrons neural network case study, varying a wide range of parameters, such as number of inputs/outputs, length of input/output data, number of neurons and layers. Experimental results show time savings up to 40% in multiple thread execution.
Research on structural dynamics in Chinese automobile standard citation network
Tập 32 - Trang 31-39 - 2018
China, who owns the largest automobile consuming market, is becoming the largest automobile manufacturing country. However, a gap still lies between China and other traditional automobile manufacturing countries in terms of design capability and production efficiency. Standardization can effectively promote technological innovation and industrial upgradation, which enhances the overall performance of the automobile industry. This paper aims at identifying the structural problem in the automobile standard citation network in China, since the citation relationship reflects the transmission and development of knowledge or technologies. To this end, a dynamic standard citation network model is developed for ease of extraction of standard data at any time points. A set of complex network metrics at both node and network level are chosen with rational explanations in the context of automobile industry. With the data collected from publicized Web sites, the topological evolution of this network is analyzed as well. We significantly show that the standard citation networks at different time periods are generally loosely connected and contain too many isolated nodes. Meanwhile, the critical nodes in the standard citation network also change dynamically. We suggest that these isolated standards should be integrated into the citation network through revision activities.
Convolutional neural networks for segmenting xylem vessels in stained cross-sectional images
Tập 32 - Trang 17927-17939 - 2019
Xylem is a vascular tissue that conducts sap (water and dissolved minerals) from the roots to the rest of the plant while providing physical support and resources. Sap is conducted within dead hollow cells (called vessels in flowering plants) arranged to form long pipes. Once formed, vessels do not change their structure and last from years to millennia. Vessels’ configuration (size, abundance, and spatial pattern) constitutes a record of the plant–environment relationship, and therefore, a tool for monitoring responses at the plant and ecosystem level. This information can be extracted through quantitative anatomy; however, the effort to identify and measure hundreds of thousands of conductive cells is an inconvenience to the progress needed to have solid assessments of the anatomical–environment relationship. In this paper, we propose an automatic methodology based on convolutional neural networks to segment xylem vessels. It includes a post-processing stage based on the use of redundant information to improve the performance of the outcome and make it useful in different sample configurations. Three different neural networks were tested obtaining similar results (pixel accuracy about 90%), which indicates that the methodology can be effectively used for segmentation of xylem vessels into images with non-homogeneous variations of illumination. The development of accurate automatic tools using CNNs would reduce the entry barriers associated with quantitative xylem anatomy expanding the use of this technique by the scientific community.
Optimal feature selection using distance-based discrete firefly algorithm with mutual information criterion
Tập 28 - Trang 2795-2808 - 2016
In this paper, we investigate feature subset selection problem by a new self-adaptive firefly algorithm (FA), which is denoted as DbFAFS. In classical FA, it uses constant control parameters to solve different problems, which results in the premature of FA and the fireflies to be trapped in local regions without potential ability to explore new search space. To conquer the drawbacks of FA, we introduce two novel parameter selection strategies involving the dynamical regulation of the light absorption coefficient and the randomization control parameter. Additionally, as an important issue of feature subset selection problem, the objective function has a great effect on the selection of features. In this paper, we propose a criterion based on mutual information, and the criterion can not only measure the correlation between two features selected by a firefly but also determine the emendation of features among the achieved feature subset. The proposed approach is compared with differential evolution, genetic algorithm, and two versions of particle swarm optimization algorithm on several benchmark datasets. The results demonstrate that the proposed DbFAFS is efficient and competitive in both classification accuracy and computational performance.
PI controller design for MPPT of photovoltaic system supplying SRM via BAT search algorithm
Tập 28 - Trang 651-667 - 2015
Maximum power point tracking (MPPT) is used in photovoltaic (PV) systems to maximize its output power. This paper introduces a new MPPT control design to PV system supplied switched reluctance motor (SRM) based on PI controller. The developed PI controller is used to reach MPPT by monitoring the voltage and current of the PV array and adjusting the duty cycle of the DC/DC converter. The design task of MPPT is formulated as an optimization problem which is solved by BAT algorithm to search for optimal parameters of PI controller. Simulation results have shown the validity of the suggested technique in delivering MPPT to SRM under atmospheric conditions. Also, the performance of the developed BAT algorithm is compared with particle swarm optimization for various disturbances to confirm its robustness.
SCCRNet: a framework for source camera identification on digital images
Tập 36 - Trang 1167-1179 - 2023
Identifying the source of digital images is a critical task in digital image forensics. A novel architecture is proposed using a combination of Convolutional layers and residual blocks to distinguish source cameras. The network architecture comprises convolutional layers, residual blocks, batch normalization layers, a fully connected layer and a softmax layer. Architecture aids in learning and extracting the features for identifying the model and sensor level patterns for source camera identification. Multiple patches are taken from each image to increase the sample space size. The experiments on the MICHE-I dataset show an accuracy of 99.47% for model level source camera identification and 96.03% for sensor level identification. Thus, the proposed method is more accurate than the state-of-the-art methods on the MICHE-1 dataset. The proposed architecture yields comparable results on Dresden and VISION datasets also. Moreover, a technique is also proposed to identify the images of unknown camera models by setting a threshold value for the output prediction score.
Similarity based person re-identification for multi-object tracking using deep Siamese network
Tập 34 - Trang 18171-18182 - 2022
The process of object tracking involves consistently identifying each instance across frames depending on initial set of object detection(s). Moreover, in multiple object tracking (MOT), the process through tracking-by-detection paradigm consists of performing two common steps consecutively, which are detection and data association. In MOT, it is targeted to associate detections across frames by localizing and identifying all objects of interest. MOT algorithms further keep tracking even the most challenging issues such as revisiting the same view, missing detections, occlusion and temporarily unseen objects, same-appearance objects coexisting in the same frame occur. Hence, re-identification (re-id) appears to be the most powerful tool for assigning the correct identities to each individual instance when aforementioned issues arise. In this work, we propose a similarity-based person re-id framework, called SAT, using a Siamese neural network via shared weights. Once detections are obtained from the backbone SAT applies a Siamese feature extraction model and then we introduce a similarity array for assessing tracklet(s) and detection(s). We examine the performance of SAT on several benchmarks with extensive experiments and statistical tests, where we improve the current state-of-the-art according to commonly used performance metrics with higher accuracy, less ID switches, less false positive and negative rates.