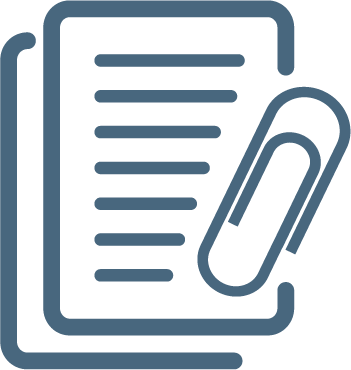
Interdisciplinary Sciences: Computational Life Sciences
1867-1462
Cơ quản chủ quản: Springer Verlag , Springer Heidelberg
Lĩnh vực:
Health InformaticsBiochemistry, Genetics and Molecular Biology (miscellaneous)Computer Science Applications
Phân tích ảnh hưởng
Thông tin về tạp chí
Các bài báo tiêu biểu
Computational approaches to identify common subunit vaccine candidates against bacterial meningitis
Tập 5 - Trang 155-164 - 2013
Bacterial meningitis, an infection of the membranes (meninges) and cerebrospinal fluid (CSF) surrounding the brain and spinal cord, is a major cause of death and disability all over the world. From perinatal period to adult, four common organisms responsible for most of the bacterial meningitis are Streptococcus pneumonia, Neisseria meningitidis, Haemophilus influenza and Staphylococcus aureus. As the disease is caused by more organisms, currently available vaccines for bacterial meningitis are specific and restricted to some of the serogroups or serotypes of each bacterium. In an effort to design common vaccine against bacterial meningitis, proteomes of the four pathogens were compared to extract seven common surface exposed ABC transporter proteins. Pro-Pred server was used to investigate the seven surface exposed proteins for promiscuous T-cell epitopes prediction. Predicted 22 T-cell epitopes were validated through published positive control, SYFPEITHI and immune epitope database to reduce the epitope dataset into seven. T-cell epitope 162-FMILPIFNV-170 of spermidine/putrescine ABC transporter permease (potH) protein was conserved across the four selected pathogens of bacterial meningitis. Hence, structural analysis was extended for epitope 162-FMILPIFNV-170. Crystal structures of HLA-DRB alleles were retrieved and structure of potH was modeled using Prime v3.0 for structural analysis. Computational docking of HLA-DRB alleles and epitope 162-FMILPIFNV-170 of potH was performed using Glide v5.7. RMSD and RMSF of simulation studies were analyzed by Desmond v3.2. The docking and simulation results revealed that the HLA-DRB-epitope complex was stable with interaction repressive function of HLA. Thus, the epitope would be ideal candidate for T-cell driven subunit vaccine design against bacterial meningitis.
Towards predictive stochastic dynamical modeling of cancer genesis and progression
Tập 2 Số 2 - Trang 140-144 - 2010
Based on an innovative endogenous network hypothesis on cancer genesis and progression we have been working towards a quantitative cancer theory along the systems biology perspective. Here we give a brief report on our progress and illustrate that combing ideas from evolutionary and molecular biology, mathematics, engineering, and physics, such quantitative approach is feasible.
Prediction of Phenotypic Effects of Variants Observed in LOC_Os04g36720 of FRO1 Gene in Rice (Oryza sativa L.)
Tập 9 Số 2 - Trang 304-308 - 2017
AC-Caps: Attention Based Capsule Network for Predicting RBP Binding Sites of LncRNA
- 2020
Long non-coding RNA(lncRNA) is one of the non-coding RNAs longer than 200 nucleotides and it has no protein encoding function. LncRNA plays a key role in many biological processes. Studying the RNA-binding protein (RBP) binding sites on the lncRNA chain helps to reveal epigenetic and post-transcriptional mechanisms, to explore the physiological and pathological processes of cancer, and to discover new therapeutic breakthroughs. To improve the recognition rate of RBP binding sites and reduce the experimental time and cost, many calculation methods based on domain knowledge to predict RBP binding sites have emerged. However, these prediction methods are independent of nucleotides and do not take into account nucleotide statistics. In this paper, we use a high-order statistical-based encoding scheme, then the encoded lncRNA sequences are fed into a hybrid deep learning architecture named AC-Caps. It consists of a joint processing layer(composed of attention mechanism and convolutional neural network) and a capsule network. The AC-Caps model was evaluated using 31 independent experimental data sets from 12 lncRNA-binding proteins. In experiments, our method achieves excellent performance, with an average area under the curve (AUC) of 0.967 and an average accuracy (ACC) of 92.5%, which are 0.014, 2.3%, 0.261, 28.9%, 0.189, and 21.8% higher than HOCCNNLB, iDeepS, and DeepBind, respectively. The results show that the AC-Caps method can reliably process the large-scale RBP binding site data on the lncRNA chain, and the prediction performance is better than existing deep-learning models. The source code of AC-Caps and the datasets used in this paper are available at
https://github.com/JinmiaoS/AC-Caps
.
Inferring Gene Regulatory Networks Using the Improved Markov Blanket Discovery Algorithm
Tập 14 Số 1 - Trang 168-181 - 2022
Using Network Distance Analysis to Predict lncRNA–miRNA Interactions
Tập 13 Số 3 - Trang 535-545 - 2021
A computational model for genetic and epigenetic signals in colon cancer
Tập 5 - Trang 175-186 - 2013
Cancer, a class of diseases, characterized by abnormal cell growth, has one of the highest overall death rates world-wide. Its development has been linked to aberrant genetic and epigenetic events, affecting the regulation of key genes that control cellular mechanisms. However, a major issue in cancer research is the lack of precise information on tumour pathways; therefore, the delineation of these and of the processes underlying disease proliferation is an important area of investigation. A computational approach to modelling malignant system events can help to improve understanding likely “triggers”, i.e. initiating abnormal micro-molecular signals that occur during cancer development. Here, we introduce a network-based model for genetic and epigenetic events observed at different stages of colon cancer, with a focus on the gene relationships and tumour pathways. Additionally, we describe a case study on tumour progression recorded for two gene networks on colon cancer, carcinoma in situ. Our results to date showed that tumour progression rate is higher for a small, closely-associated network of genes than for a larger, less-connected set; thus, disease development depends on assessment of network properties. The current work aims to provide improved insight on the way in which aberrant modifications characterize cancer initiation and progression. The framework dynamics are described in terms of interdependencies between three main layers: genetic and epigenetic events, gene relationships and cancer stage levels.
Electromagnetic detection of HIV DNA in the blood of AIDS patients treated by antiretroviral therapy
Tập 1 - Trang 245-253 - 2009
Electromagnetic signals of low frequency have been shown to be durably produced in aqueous dilutions of the Human Imunodeficiency Virus DNA. In vivo, HIV DNA signals are detected only in patients previously treated by antiretroviral therapy and having no detectable viral RNA copies in their blood. We suggest that the treatment of AIDS patients pushes the virus towards a new mode of replication implying only DNA, thus forming a reservoir insensitive to retroviral inhibitors. Implications for new approaches aimed at eradicating HIV infection are discussed.
Identifying a Gene Knockout Strategy Using a Hybrid of Simple Constrained Artificial Bee Colony Algorithm and Flux Balance Analysis to Enhance the Production of Succinate and Lactate in Escherichia Coli
Tập 11 - Trang 33-44 - 2019
In recent years, metabolic engineering has gained central attention in numerous fields of science because of its capability to manipulate metabolic pathways in enhancing the expression of target phenotypes. Due to this, many computational approaches that perform genetic manipulation have been developed in the computational biology field. In metabolic engineering, conventional methods have been utilized to upgrade the generation of lactate and succinate in E. coli, although the yields produced are usually way below their theoretical maxima. To overcome the drawbacks of such conventional methods, development of hybrid algorithm is introduced to obtain an optimal solution by proposing a gene knockout strategy in E. coli which is able to improve the production of lactate and succinate. The objective function of the hybrid algorithm is optimized using a swarm intelligence optimization algorithm and a Simple Constrained Artificial Bee Colony (SCABC) algorithm. The results maximize the production of lactate and succinate by resembling the gene knockout in E. coli. The Flux Balance Analysis (FBA) is integrated in a hybrid algorithm to evaluate the growth rate of E. coli as well as the productions of lactate and succinate. This results in the identification of a gene knockout list that contributes to maximizing the production of lactate and succinate in E. coli.