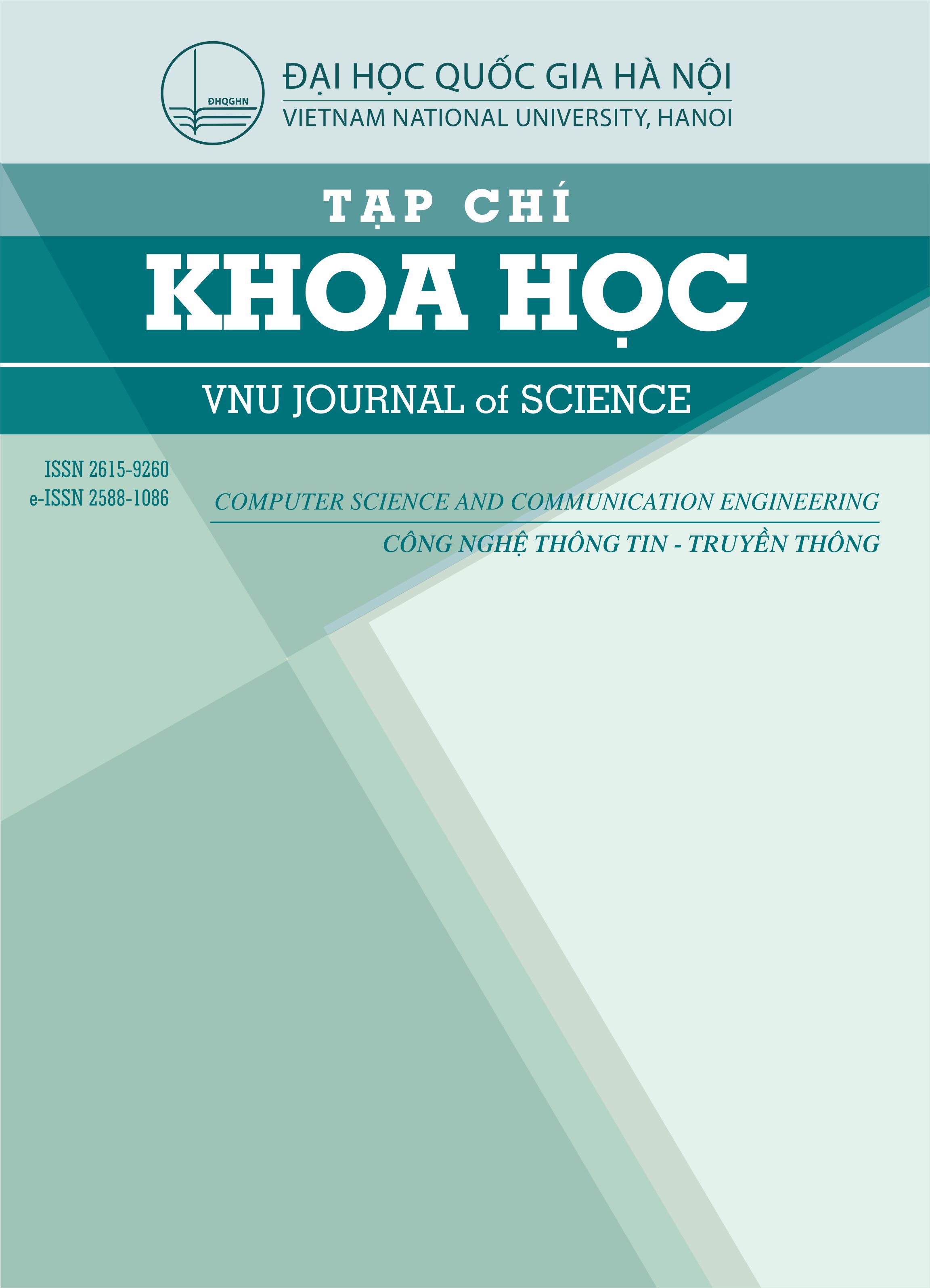
VNU Journal of Science: Computer Science and Communication Engineering
2615-9260
Việt Nam
Cơ quản chủ quản: N/A
Lĩnh vực:
Các bài báo tiêu biểu
Deep Learning for Epileptic Spike Detection
Tập 33 Số 2 - 2018
In the clinical diagnosis of epilepsy using electroencephalogram (EEG) data, an accurate automatic epileptic spikes detection system is highly useful and meaningful in that the conventional manual process is not only very tedious and time-consuming, but also subjective since it depends on the knowledge and experience of the doctors. In this paper, motivated by significant advantages an...... hiện toàn bộ
Human Action Recognition Using Dynamic Time Warping and Voting Algorithm
Tập 30 Số 3 - 2014
This paper presents a human action recognition method using Dynamic Time Warping and voting algorithms on 3D human skeletal models. In this method, human actions which are the combinations of multiple body part movements are described by feature matrices in concerning with both spatial and temporal domains. The feature matrices are created based on the spatial selection of time series of relative ...... hiện toàn bộ
A Big Data Analytics Framework for IoT Applications in the Cloud
Tập 31 Số 2 - 2015
The Internet of Things (IoT) is an evolution of connected networks including million chatty embedded devices. A huge amount of data generated day by day by things must be aggregated and analyzed with technologies of the "Big Data Analytics". It requires coordination of complex components deployed both on premises and Cloud platforms. This article proposes BDAaaS, a flexibly adaptive cloud-based fr...... hiện toàn bộ
Max – Min Composition of Linguistic Intuitionistic Fuzzy Relations and Application in Medical Diagnosis
Tập 30 Số 4 - 2015
In this paper, we first introduce notion of linguistic intuitionistic fuzzy relations. This notion may be useful in situations when each correspondence of objects is presented as two labels such that the first expresses degree of membership, and the second expresses degree of non-membership as in the intuitionistic fuzzy theory. Sanchez's approach for medical diagnosis is extended using linguistic...... hiện toàn bộ
Performance Analysis of Cooperative-based Multi-hop Transmission Protocols in Underlay Cognitive Radio with Hardware Impairment
Tập 31 Số 2 - 2015
In this paper, we study performances of multi-hop transmission protocols in underlay cognitive radio (CR) networks under impact of transceiver hardware impairment. In the considered protocols, cooperative communication is used to enhance reliability of data transmission at each hop on an established route between a secondary source and a secondary destination. For performance evaluation, we der...... hiện toàn bộ
VLSP 2021-ViMRC Challenge: Vietnamese Machine Reading Comprehension
Tập 38 Số 2 - 2022
One of the emerging research trends in natural language understanding is machine reading comprehension (MRC) which is the task to find answers to human questions based on textual data. While many datasets have been developed for MRC research for other languages, there is a lack of such resources for the Vietnamese language. Although many datasets and methodologies have been developed for English a...... hiện toàn bộ
A Novel Combination of Negative and Positive Selection in Artificial Immune Systems
Tập 31 Số 1 - 2015
Artificial Immune System (AIS) is a multidisciplinary research area that combines the principles of immunologyand computation. Negative Selection Algorithms (NSA) is one of the most popular models of AIS mainly designed for one-class learning problems such as anomaly detection. Positive Selection Algorithms (PSA) is the twin brother of NSA with quite similar performance for AIS. Both NSAs and ...... hiện toàn bộ
Liver Segmentation on a Variety of Computed Tomography (CT) Images Based on Convolutional Neural Networks Combined with Connected Components
Tập 36 Số 1 - 2020
Liver segmentation is relevant for several clinical applications. Automatic liver segmentation using convolutional neural networks (CNNs) has been recently investigated. In this paper, we propose a new approach of combining a largest connected component (LCC) algorithm, as a post-processing step, with CNN approaches to improve liver segmentation accuracy. Specifically, in this study, the algorithm...... hiện toàn bộ
An Adaptive and High Coding Rate Soft Error Correction Method in Network-on-Chips
Tập 35 Số 1 - 2019
The soft error rates per single-bit due to alpha particles in sub-micron technology is expectedly reducedas the feature size is shrinking. On the other hand, the complexity and density of integrated systems are accelerating which demand ecient soft error protection mechanisms, especially for on-chip communication. Using soft error protection method has to satisfy tight requirements for the area an...... hiện toàn bộ