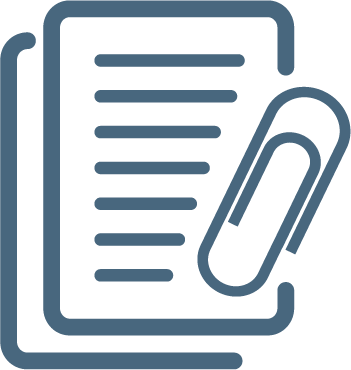
Journal of Statistical Software
SCIE-ISI SCOPUS (1996-2023)
1548-7660
1548-7660
Mỹ
Cơ quản chủ quản: JOURNAL STATISTICAL SOFTWARE , University of California at, Los Angeles
SCIE-ISI SCOPUS (1996-2023)
1548-7660
1548-7660
Mỹ
Cơ quản chủ quản: JOURNAL STATISTICAL SOFTWARE , University of California at, Los Angeles